Project Kick-off: 1st of April 2022
Milestone 1: Midterm Review; 11th of July 2022
Milestone 2: Midterm review: 12th of October 2022
Milestone 3: Final Presentation / Review: 11th May 2023
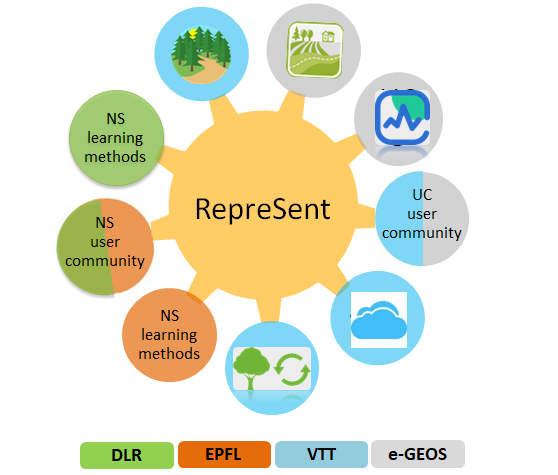
• Five EO UCs are proposed that covers a variety of locations (Northern Europe, Southern Europe, Africa), spanning over large spatial and temporal extents (regional coverage, long time series) and widely exploiting Sentinel-1, Sentinel-2, and Landsat archives.
• UCs focus on EO challenges where either labelled data are scarce (e.g., anomaly detection in ground deformation analysis) or the successful application of supervised methods requires many labels that are tedious or costly to collect (e.g., detailed land cover/land cover change mapping, forest disturbance monitoring, cloud detection/removal).
• The EO UCs are tackling core business and scientific questions since they are tightly connected to e-GEOS and VTT core business areas and active projects. This ensures also a close link to users and stakeholders in the different sectors.
• Comparisons will be provided to suitable baselines to quantitatively understand whether non-labelled data helps to improve the performance.
• Computation requirement with respect to training time, test time, any hardware requirement, required number of images (unlabelled) will be reported.
• As per suitability to the use cases, a number of non-supervised learning methods will be tested, including self-supervised methods (e.g., deep clustering and contrastive learning), unsupervised methods (e.g., deep change vector analysis, multi-temporal segmentation, and temporal anomaly detection), and meta learning.
• A 1-day workshop is planned to be organized as part of the project.
• The consortium proposed a scientific session to the ESA Living Planet Symposium 2022.
• Two networking sessions will be organized with the European EO start-ups.
• The results will be promoted at different conferences/workshops and results will be published in appropriate journals. Contact will be established with the research groups addressing similar or related thematic areas in the AI/EO/AI4EO community.
• The project results will be disseminated via the project website, e-mails, and social media.